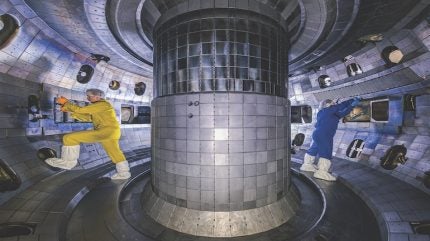
While major breakthroughs put the realisation of nuclear fusion nearer than ever, a major challenge to developing the technology as a commercial reality remains plasma control in magnetic confinement devices. The chaotic behaviour of plasma in tokamak designs is a major barrier to reliably creating efficient fusion reactions and the ability to harness its energy effectively.
Now though, Luxembourg-based Next Step Fusion has demonstrated a new approach to plasma control using artificial intelligence.
Aleksei Zolotarev, CEO & Founder of Next Step Fusion, explains the limitations of the majority of the current plasma control strategies in use today, telling Nei: “Normally, Conventional control systems use magnetic data in real-time to respond to changes in the magnetic system. While this method is reliable and commonly used, it often depends on deterministic algorithms that can be slow to adjust to unexpected situations. Machine learning improves control by using predictive and adaptive strategies. It can handle uncertainties in the system, making the control process more flexible and able to maintain performance even when conditions change. Machine learning allows these boundaries of the controls to broaden out because they can include some sort of remediation to address uncertainty in the process and thus be more sustainable in the face of some deviations from the initial strategy or from the established target conditions.
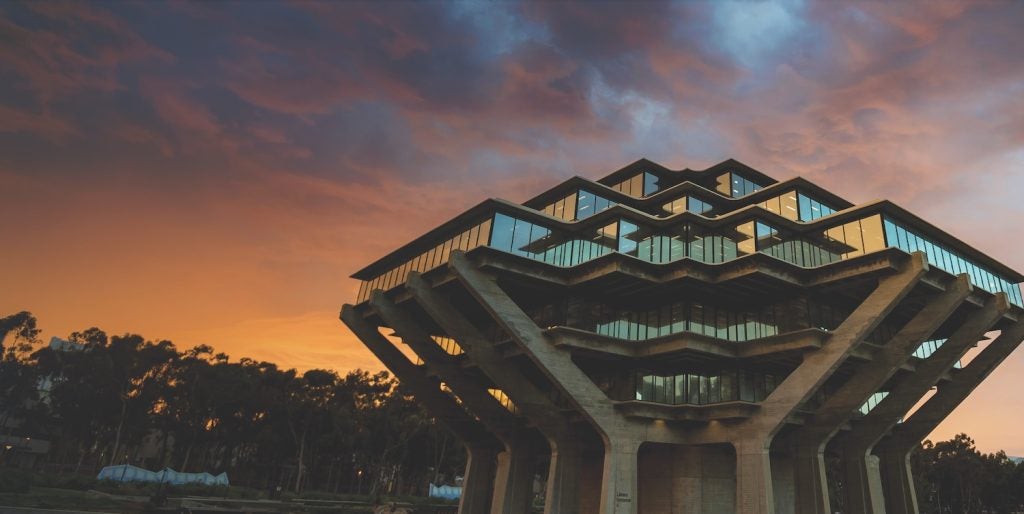
“To provide a robust and sustainable control system for the machine, every case must be foreseen, and every potential pitfall must be addressed in the operating strategy. In conventional machine learning control, you usually build a specific dataset and use previous experimental data for training the agent to show it exactly which scenarios were good and which are bad. It then learns how to distinguish one from another and tries to follow the best case.”
He goes on to set out the reinforcement learning concept to train a plasma control machine learning model: “What is different from the conventional machine learning approach is the application of reinforcement learning. In our approach we just drop the agent into the environment using a digital replica of the device and its hardware with the physics included. Our control agent is trained not on pre-set data but in a digital environment that simulates tokamak behaviour very precisely. The agent then randomly starts to study this environment and a subsystem then scores the results of the actions of the agent. It learns how to score best in this environment and in this process, the agent learns the policy for control rather than an identification method. This is what we call reinforcement learning.”
Although Zolotarev readily acknowledges some peers have used the AI plasma control model previously –notably DeepMind’s success in controlling plasma using reinforcement learning on the mid-sized Tokamak à Configuration Variable (TCV) tokamak in Switzerland – Next Step Fusion have demonstrated this approach at scale. The company has been conducting experiments at the DIII-D National Fusion Facility tokamak in San Diego, California, together with partners the University of California San Diego. The DIII-D tokamak is nearly double the size of the TCV device.
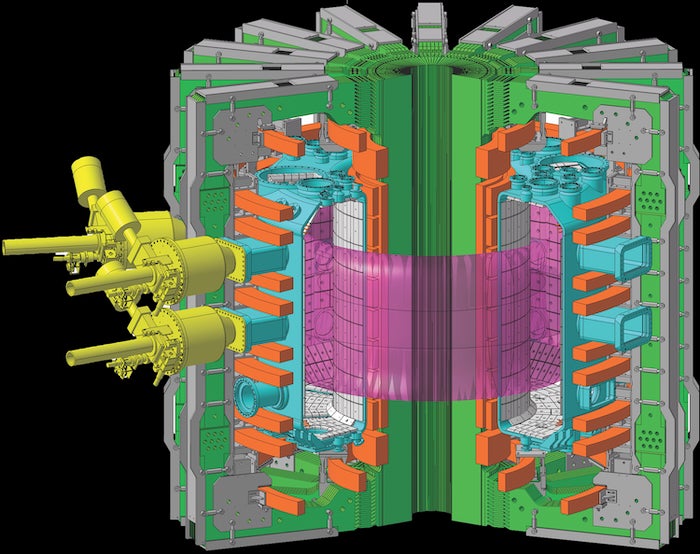
Scaling up AI control
On the DIII-D device sensors detect the magnetic field which is used as the input for the learning model which simply aims to optimise field performance rather than attempt to optimise the magnetic field characteristics around a pre-defined pattern.
Zolotarev says: “This experiment was only about controlling the small poloidal coil system of the DIII-D machine using the raw magnetic diagnostic sensor data. We don’t use any additional transformations of this data, taking raw magnetic data from sensors directly and the output delivers control system commands for the magnetic coils. This approach doesn’t necessarily require experimental data to train the model, but the model demonstrates good performance with experimental data in real-time”.
Next Step Fusion scientists created a digital twin replica of the DIII-D device to train and test their machine learning models. “We recreate the whole machine, its geometry, magnetic system, passive and active conductive elements, and then tell agent to use this environment to find the optimal policy for control. As soon as agent has made millions of experiments, then we transfer this agent to the real machine in this case with this DIII-D tokamak. You use experimental data to improve the model, like a conventional AI, but we don’t use a real machine, instead we use its digital replica which we created using our simulator,” Zolotarev adds.
The experimental data shows that the model produces better characteristics for setting plasma control. “We always refine our tools against real experimental data or against a simulator that is native for this DIII-D tokamak to try validate our models and see how precise we are against real data. That allows us to keep the precision, the accuracy, on each step. Otherwise, you could end up with a small discrepancy on each step and then end up being very, very inaccurate,” notes Zolotarev.
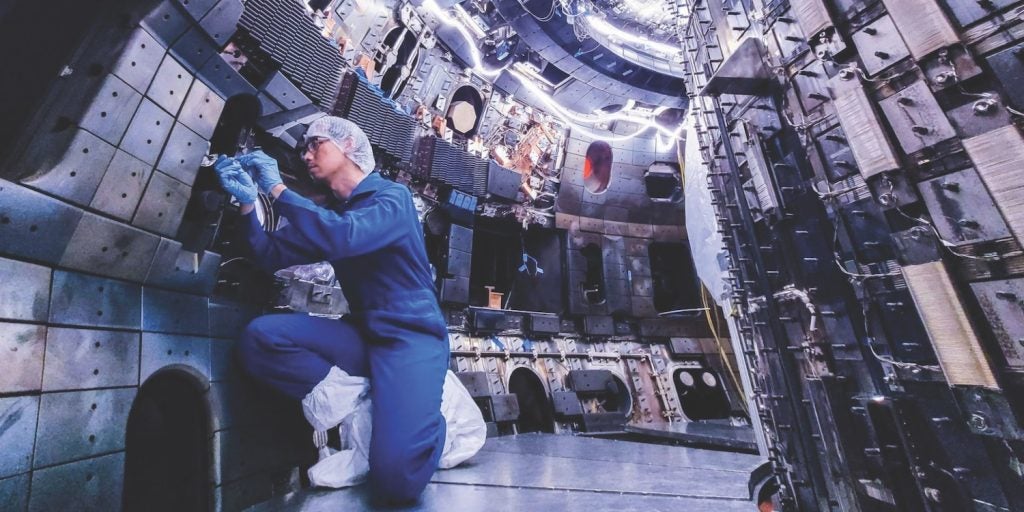
The AI is dynamic and controls the magnetic field in real time as the fusion experiments take place. However, their simulator, called NFSsim, which is doing a lot of number crunching while learning, is actually slower than the real physics in the tokamak itself.
Nonetheless, the control model which emerges is operating in real time. Zolotarev explains: “The simulation provides us with the evolution of the environment through time. It’s not in real time, it’s a bit slower, but it’s fast enough to be used in reinforcement learning. This is an important consideration because reinforcement learning requires numerous starts of the environment and numerous calculations of the environmental evolution. During the training, you can include some instabilities and deviations from initial conditions and make the agent robust to many things that appear in real life experiments. The control agent is made small enough and fast enough to be included in the real time control loop”. He continues: “When you integrate the agent with the real tokamak, it needs to be the same speed as the current tokamak system, which is a few thousands of operations per second. That was one of the challenges in integrating our agent into this fast environment and to convince the DIII-D team that our plasma control model is fast enough and robust enough, because you don’t want to fail the whole plasma control system with the new module”.
Indeed, the simulation was subsequently validated using real experimental data and the GSevolve simulator (DIII-D) to ensure its accuracy and reliability. As Zolotarev says, the new model represents a substantial improvement. “Normally to control the magnetic field they use so-called feedforward controls. They create a pre-scripted scenario on how the currents on the magnetic coils change over time and once the experiment begins that process is executed. There are also feedback controls, but it’s not that smart and is mostly based on PID controllers”. PID controllers work well for basic control tasks, but they can’t predict system behaviour or adapt dynamically. Machine learning can improve control and adaptability for complex plasma conditions in tokamaks”.
To date, nine successful plasma discharges on the DIII-D tokamak have confirmed the effectiveness of their model. “We successfully kept the plasma stable during several discharges on the DIII-D tokamak and we gained valuable insights for further tokamak control and performance optimisation,” said Zolotarev. However, in terms of the specific performance improvement from the model Zolotarev is clear that the project is still at the research and analysis phase. He says: “Claiming some improvement is one of the things where we want to be peer-reviewed and to have a scientifically-proven result. What we do observe is that our agent survives the operations in different modes. There are different modes of confinement in tokamaks such as L-mode and H-mode [high and low confinement] and our machine learning agent also managed the important H-L transition without losing control of the plasma’s position. These results show that our controller performs strongly on such a large scale in real-world tokamaks. This is important because this is something that may happen in future fusion reactors and power plants”.
Creating a generic control model
Georgy Subbotin, CTO at Next Step Fusion, points to the future development of reinforcement learning as a route to a generic magnetic field control system for a whole class of fusion devices. He says: “We have been working on this machine learning application for fusion control for almost a year and a half and we believe that we have made a first great step. We have also put a pipeline in place so we can train this model for different tokamaks and we can do it relatively quickly so it will not take another one and a half years to train for the next machine. It will take a few weeks. We are ready to scale these results on other devices and we actually have a couple of tokamaks in mind where we have agreed to test our machine learning technology”.
As for how much time it will take to create, an operational plasma control system based on machine learning, the Next Step Fusion team indicate it will take several more years but important progress has already been demonstrated. “We believe that at the moment we have demonstrated with the agent that reinforcement learning is definitely the right approach to apply. But we need to scale our approach to control not only of the magnetic system, but also the heating system and on the disruption prediction system and other subsystems of tokamaks, which are essential for future power producing reactors,” says Subbotin.
Subbotin continues: “We understand that in some of these niche areas like disruption prediction, there is already progress being made by scientific institutions and other groups but in other areas, there is no such progress and we believe we will be the first to apply reinforcement learning to some of these problems. We believe that we will be able to solve them and build a holistic solution for plasma control, which could be applied not only on the current generation of scientific tokamaks, but also on future machines, which will be closer to energy producing reactors. That’s the challenge, because reactor-relevant devices will differ greatly from the research facilities where we work now. In future we want to be working on energy producing reactors”.
The ultimate goal then is to develop a generic model that will apply to any tokamak-type machine, but will also include other magnetic confinement fusion devices.
Zolotarev explains: “The plasma control system which we intend to build will cover magnetic confinement devices – tokamaks and stellarators – because we believe that, although many stellarator companies claim that their control will be much easier than with tokamaks, they still need high quality software to control the processes. They don’t need complex control for a magnetic system, perhaps, but they still need to control heating, the density, and energy production in general. It will still require sophisticated algorithms to coordinate different subsystems of the device and its plasma control system. We believe that the principles which we put into our control system will allow us to cover both tokamaks and stellarators”.
A future of AI-informed design
Looking even further ahead, AI systems developed on the basis of reinforcement learning may also inform the core design of future commercial fusion devices. “When you design a device, you definitely take into account the capabilities of the control system and even the coils. For example, in-vessel coils depend on how stable plasma conditions are without them and you definitely don’t want to have anything inside the vacuum vessel if possible. It’s also beneficial that this approach can aim for optimisation of control for higher-level targets, like energy production or something else that is a result of combinations of different parameters. Assuming that you can optimise the position of coils, for example, or heating systems, with regard to capabilities of your control system it potentially can inform the design, but we’re not there yet,” says Zolotarev. Nonetheless, he argues that now is the right moment to discuss future tokamak designs in the context of AI in control and simulation systems. And he envisages an even greater role for AI in the fusion power plant designs of the future. “What we want to do is to automate it somehow using tools that take assumptions from the control strategies and inform the design. Imagine you are able to generate 1,000 tokamaks of slightly different designs then run some calculations for each of these designs and see which one works better, where control tends to be more stable. That’s the strategy,” he says.
Given these future goals, the current strategy for Next Step Fusion is to involve academic research institutions as much as possible. The company is, for example, already working on different projects with Columbia University using not only reinforcement learning, but a whole range of conventional and AI/machine learning tools.
Zolotarev concludes: “We all believe in fusion as an industry and that’s why we are focusing on control because as soon as the materials and design problems are solved, future tokamaks and fusion power plants need reliable control. Fusion devices will only be successful commercially if down times, disruptions, the time for maintenance is as low as possible. It’s one of the biggest factors affecting commercial efficiency and we believe that it all starts with control. If your control is reliable and robust, you have less down time, you damage your machine less, and you have higher plasma parameters for any given machine”.
It adds up to higher load factors and a lower lifetime cost of energy. For commercial fusion then, Next Step believes control is what counts.