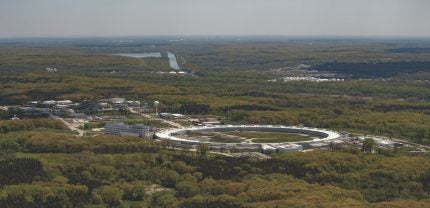
In December 2023 around 100 experts in energy, artificial intelligence (AI) and machine learning (ML) convened at Argonne National Laboratory. They were there to map out the future potential for utilisation of AI in energy, considering five ‘energy mission’ areas that have previously been identified – fossil fuel, carbon management, nuclear power, renewables and energy efficiency. The outcome is a report, ‘AI for Energy’ that sets out ‘grand challenges’ for AI applications in nuclear energy over the next decade, as well as for the power grid, carbon management, energy storage and energy materials.
The experts were optimistic: they found that AI offers the ability to assess much larger system models, make more use of computational resources and capture knowledge from top scientists. As a result, it offers a “transformational opportunity” to rapidly deploy clean energy assets, secure critical networks and reduce the capital and operational costs of next-generation energy technologies. It can also help the energy industry grapple with new issues like connected systems and demand-side response.
AI use cases across the broader energy industry are varied and perhaps sometimes unexpected. Among those noted in the report that followed the Argonne meeting were:
- Commercial power plant design and licensing, which can account for up to 50% of the time-to-market for new energy deployments. The experts said AI has the potential to reduce schedules by approximately 20% across new clean energy designs, partly by augmenting the large energy development workforce that will be required.
- Responding to a rapid increase in requirements for planning, operation and controls as demand-side response activities increase alongside generation capabilities. That encompasses more flexibility from assets and improving the reliability and agility of the grid by increasing the visibility of assets to operators and consumers. The resulting volumes of communications, controls, data and information are increasing and require an AI-based response.
- Natural disasters and human-caused events, which are occurring more frequently and with more intensity, with adverse weather events increasingly disrupting supply chains, damaging assets and making some areas less habitable. An AI-based, all-hazards global response system can ingest global and stakeholder datasets, to enhance preparedness and allow for faster recovery.
- Resource extraction and use, where science-based models that include AI multi-modelling approaches can improve predictions of subsurface properties, to help find critical material resources such as uranium, and identify features such as geothermal reservoirs or water sources. This might be realised via a national subsurface AI and data testbed. AI can also accelerate the qualification of material through automation of materials testing, to support development of new energy technologies such as advanced nuclear reactors and new batteries.
To meet the challenge of AI and take advantage of these opportunities, the experts said fundamental developments are needed.
Although US national laboratories already have hundreds of petabytes’ worth of data, so far only small amounts of these data are catalogued, warehoused and ready for AI model ingestion. The national laboratories must establish a computing ecosystem to train and host data and foundation models at increasing scales. Fine-tuned models have to be developed for each domain that are coupled, where possible, with ‘ground-truths’ (ie directly observed data) and first-principles physics. Curation of one-of-a-kind, real-world data coupled with energy industry data will be essential to building models at scale. Most important, partnerships across laboratories, government, industry, and academia are essential to realising the benefits of AI.
Implications for nuclear
In addition to these energy industry opportunities, the experts identified unique use cases in nuclear, which was named as one of five areas “vital to the energy future of the US”. (The others are the power grid, carbon management, energy storage, and energy materials.)
Their report warns that nuclear is in an AI arms race. Nuclear must embrace AI to remain competitive in the electricity generation market and attract investment, because AI is increasingly being used in competing generation technologies.
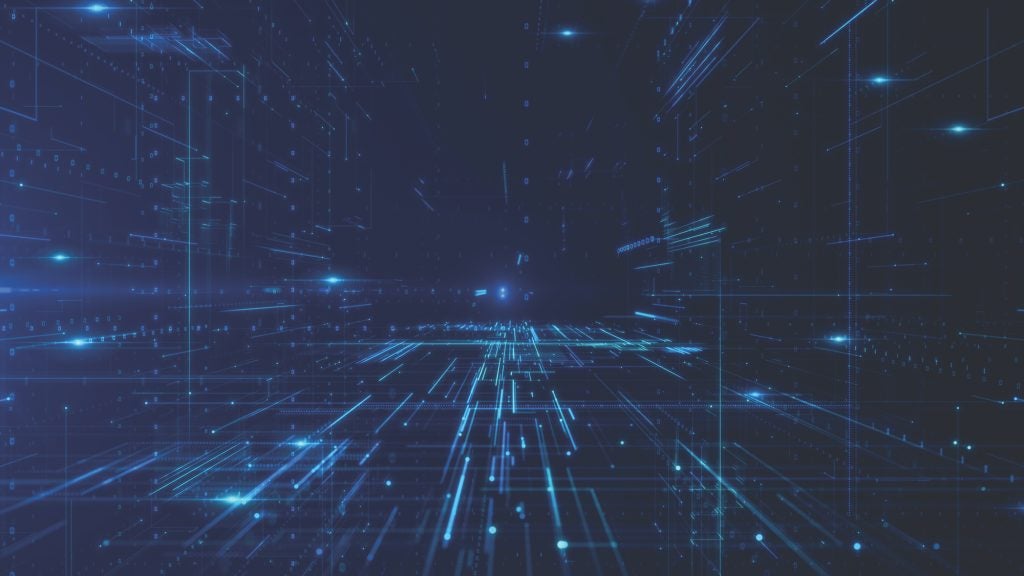
But it says nuclear can realise more benefit from AI because its intricate interdependencies pose challenges “well-suited for AI solutions”, in particular AI’s knowledge capture and capability to discern cross-disciplinary connections.
AI can also maintain expertise over time. In its assessment the report notes that during its lifecycle a nuclear plant will be the focus of different activities that involve different skill sets, most multidisciplinary, and different people – such as moving from construction to operation. That can be costly: one reason for delays and cost overruns in reactor projects resulted from construction beginning before the design was completed. The report says, “These interdependencies and the challenges that they present are eminently addressable using AI,” because AI “offers an essentially limitless capability to store knowledge and the ability to recognise connections across disciplines where subject matter experts are inherently limited”.
The report highlights three specific ‘grand challenges’ where it says AI/ML can outperform human teams in nuclear: streamlining the licensing and regulatory process; accelerating deployment; and facilitating unattended operation.
Licensing
The report describes the US regulatory process as “slow, expensive and convoluted”, especially for nuclear. From design and construction to operations and eventual decommissioning, “every phase of a nuclear reactor project undergoes rigorous scrutiny from the regulator”. The process to obtain a construction permit and operating licence for a new reactor is at least five years – sometimes running into decades when pre-application engagement is taken into account – at a cost that can escalate to hundreds of millions of dollars. For example, in the most recent approval of a NuScale US600 the company had to invest more than $500m and two million labour-hours to prepare its licensing application. What is more, the regulatory process, which was designed for light-water reactors, may not be fit for other projects.
Emerging AI technology can streamline and expedite the nuclear regulatory licensing and compliance process and make it much more cost effective for both the licensee and the regulator (for more on this subject see ‘AI and the nuclear safety case‘, NEI, June 2024).
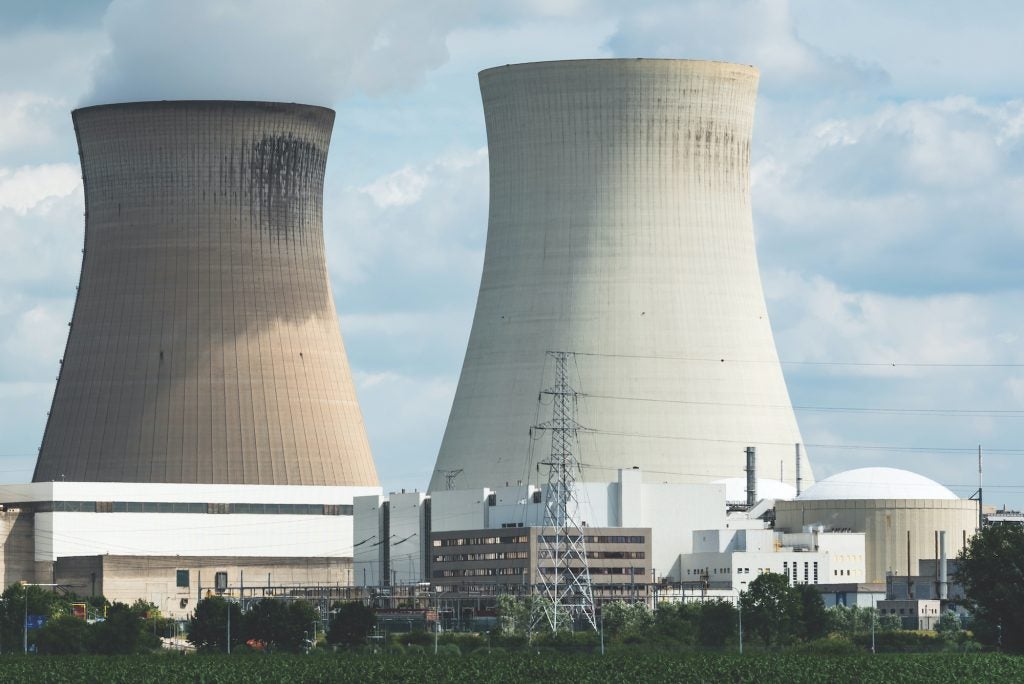
Accelerating Deployment
The siting of new energy generation capacity is a complex challenge, balancing technology options, community needs, environmental factors and resiliency considerations. But in one DOE estimate it is found that the US will need 200 GW of new nuclear generation by 2050 to meet national decarbonisation targets. It is likely that a combination of large, small, and microreactors will be used which multiplies the number of sites required.
The report says AI could aid energy planning based on a comprehensive dataset and a trained community energy foundation model that captures characteristics of (and interactions between) physical infrastructure, human behaviour and climate or weather impacts. AI can also potentially reduce future power needs by optimising site selection requirements such as the amount of water needed to operate efficiently.
Generative AI and digital engineering technologies (digital tools and software used to design, build, and analyse engineering systems) could also dramatically reduce the time and cost before construction and reduce the probability of errors during the construction phase.
The report suggests AI models could generate the outlines, descriptions and key artifacts needed early in the nuclear power plant development lifecycle to support environmental, stakeholder and engineering document development. This output can be validated with existing tools to provide a cross-check on the physical reasonableness of an AI-generated design.
A study of digital engineering and digital twinning applied to nuclear power plant design and construction found a 21% reduction in the probability of schedule delays. If 200 GW of nuclear is needed, and the report cites estimates that a “well-executed first-of-a-kind nuclear construction project is around $6,200 per kW”, reducing delays by 21%, potentially saves hundreds of billions of US energy development dollars by 2050.
Autonomous operation
A modern 1100 MW gas-fired power plant has 35 employees, while a comparably sized nuclear site requires 800 people. The report says AI can substitute for human presence across a wide range of tasks in a nuclear plant, reducing this cost disadvantage.
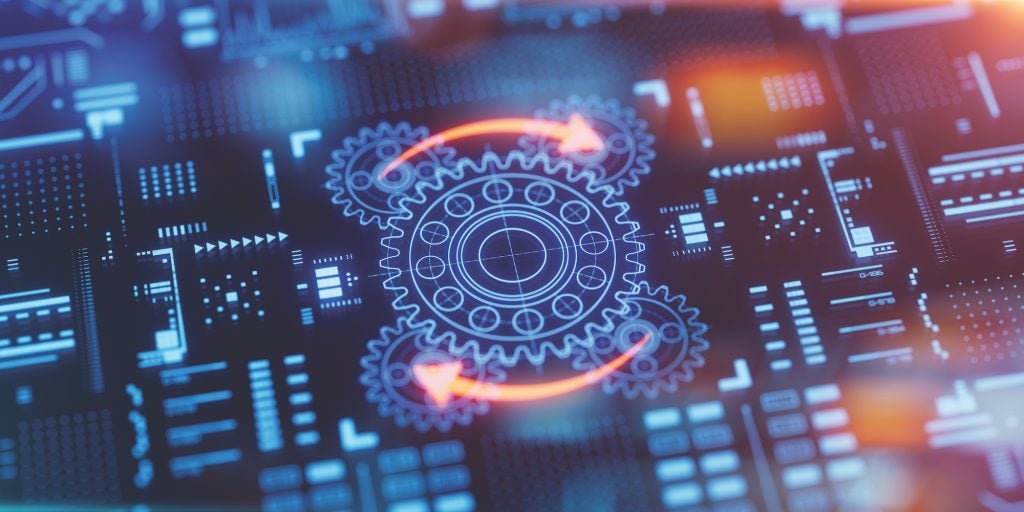
AI will minimise the need for direct human involvement by simultaneously carrying out complex cognitive tasks involving many engineering disciplines. Further, the report says the objective should be to move toward semi-autonomous operation and maintenance, because new advanced nuclear technologies, such as microreactors, will have to operate autonomously to realise economies of scale.
Of course, any unattended operation of nuclear reactors raises safety considerations; however, many plant-level digital twins of piping, valve, heat exchanger and cooling towers could be shared across applied energy domains.
On the positive side, operating in an unattended mode makes it possible to coordinate and manage monitoring, control and maintenance activities for several plants from a single remote centre. In this mode, the AI performs lower-level tasks locally at the plant. Higher-level tasks are outsourced to the remote centre where the results of the lower-level tasks provide input to tasks for maintenance scheduling and supply chain management.
In this autonomous regime, AI can also analyse large amounts of sensor and other plant data that may signal potential problems. Physical maintenance is carried out in response to AI’s continuous monitoring, predicting potential malfunctions and using preventive maintenance.
The report highlights the looming shortage of qualified nuclear plant operators. The experts further say that an AI model that can capture public and private information (including restricted domain) and perform all activities over the life cycle of a nuclear plant, eventually becoming a nuclear energy expert with a knowledge base that exceeds the capabilities of a human.
Autonomous operation may also apply when meeting local and national power needs, which may involve managing and coordinating a collection of nuclear and other generating assets. Where the grid interfaces with individual generating assets, AI can help schedule those assets to ensure that electricity demand at the grid level is met at least cost and highest reliability, with collective management of generation, outages and maintenance activities. This approach improves system economics by reducing the need for reserve plant capacity.
Modern artificial intelligence approaches are designed to be able to react in unpredictable ways to create novel output. Nuclear’s requirements for safety and other industry-specific needs may require AI to be qualified for nuclear applications. Experts in AI will need to be aware of the potential threat vectors that can be used against the developed model so they can incorporate security into the design from the ground up, and also build in cybersecurity plans throughout the development lifecycle to prevent misuse of the training data and vulnerabilities in the model, as well as safe operation throughout its lifecycle.
Five actions recommended
The report makes five recommendations to allow the industry to take advantage of some of the AI opportunities it has identified:
- Develop a leadership computing capability ecosystem The report highlights the AI4E initiative, which would see an ecosystem of high-performance computing capabilities replace stand-alone individual machines, which it says are under-used. The increase in computational capability would help solve problems in nuclear energy that are presently beyond the reach of any single machine. High-speed ‘data pipes’ are needed to facilitate communication, with algorithms to coordinate tasks and to seamlessly exchange data.
- Expand on base foundational models to include nuclear energy Because the specialised domain knowledge associated with nuclear generation is not well represented in existing foundation models more needs to be dome to expand this capacity.
- Ensure that AI enabling technologies and tools are available for nuclear Current tools are application- oriented and confined to the needs of subject matter experts without regard necessarily for what a larger enabling ecosystem might look like. Nuclear energy has unique security and safety issues that are not adequately addressed by current AI environments.
- Provide for testbeds for validating and evaluating AI methods It will be necessary to train on a platform with rich scientific data sets, ideally with a physical test bed. This will be necessary because the value proposition of AI for nuclear energy will almost certainly involve improving the efficiency of plant operations and maintenance of equipment.
- Integrate existing infrastructure in a nationwide research resource A new mindset that aims to spur collaboration would better fit an environment where AI is expected to house all that has been learned about nuclear energy. In the infrastructure domain, work could start with training related systems independently. Later, when the AI infrastructure has sufficiently developed, they would be trained together.
Even before these steps are taken, it is critical to create a comprehensive data repository and an ecosystem around it. While data are abundant, databases’ formats, availability and provenance are highly inconsistent. Some are in the form of hybrid data forms with qualitative and quantitative descriptions and inhomogeneous data.
There is an additional wealth of experimental data that reaches back to the early days of the development of the peaceful use of nuclear energy. It is largely inaccessible as the data owners do not have the resources to retrieve and order it in a form ready for use but this may be an opportunity to use large language models, as has been demonstrated in the private sector.
AI has to access the output of computer codes that represent the physics of a nuclear system if it is to have its own internal and accurate representation of the physical system. It needs to be able to understand when insufficient data is provided, such as in first-of-a-kind designs. In parallel to data collection and methods development, a test platform is needed to evaluate and tune the models that can mine existing test data.
The report says the cost involved in moving to an AI-enabled industry means there will have to be early financial benefits. In the first step, it recommends four main teams to take it forward (for data, methods, testing and infrastructure) supported by functions such as legal, to ensure that data sharing is compliant with laws, establish agreements with private industry and ensure models meet industry regulations.